Understanding the Basics of Predictive Modeling
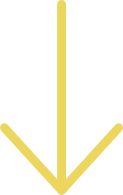
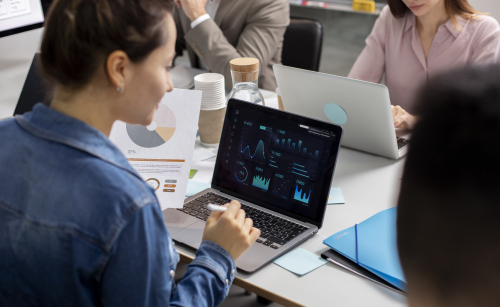
Quick Listen:
What if businesses, governments, and even hospitals could anticipate future outcomes with remarkable accuracy? That’s the promise of predictive modeling, a data-driven approach that harnesses past trends to forecast what’s coming next. Whether it’s financial markets predicting recessions, retailers anticipating inventory demand, or healthcare professionals assessing patient risks, predictive models shape decisions that affect millions.
At its core, predictive modeling is about recognizing patterns. By analyzing historical data and applying statistical techniques and machine learning algorithms, organizations gain insights that allow them to stay ahead of the curve. This technology is no longer just a tool for data scientists it has become essential for decision-makers across industries. Learn more from Heavy.AI.
The Alchemist’s Toolkit: Key Components of Predictive Modeling
Much like an alchemist transforms base metals into gold, data scientists refine raw information into actionable predictions. The process involves several key components:
- Data mining and pattern recognition: Identifying relationships within large datasets helps predict future trends. For example, an e-commerce company can forecast customer preferences based on past purchases, as explained by NetSuite.
- Risk assessment methodologies: Banks and insurance firms use predictive models to evaluate financial risks, determining credit scores and fraud probabilities, as outlined by Investopedia.
- Decision-making frameworks: Whether through traditional statistical models like regression analysis or advanced deep-learning networks, predictive analytics offers a roadmap for strategic business planning, according to IBM.
From Wall Street to Main Street: Industries Transformed
Predictive modeling is revolutionizing industries worldwide. Some of its most notable applications include:
- Finance: Banks use predictive algorithms to detect fraudulent transactions and assess creditworthiness. Hedge funds deploy machine learning to forecast stock market trends. Industry insights are provided by SAS.
- Healthcare: From diagnosing diseases to improving patient care, predictive analytics helps physicians make informed medical decisions. For example, hospitals use AI-driven models to predict readmission rates and optimize resource allocation, as detailed by Data Science Cancer.
- Retail: Online retailers personalize shopping experiences by analyzing customer behavior, optimizing inventory, and forecasting seasonal demands. Amazon’s recommendation engine, for instance, is powered by sophisticated predictive algorithms, as discussed by Google Cloud.
The Competitive Edge: Why Predictive Modeling Matters
In today’s hyper-competitive market, businesses leveraging predictive modeling gain an unmistakable advantage. The ability to anticipate shifts in consumer behavior or economic trends translates into higher efficiency, lower costs, and smarter decision-making.
Consider Netflix, which uses predictive analytics to recommend content based on viewing habits, keeping users engaged and boosting retention. Or UPS, which optimizes delivery routes using AI-powered forecasting, saving millions in fuel costs annually. Case study insights can be found on ResearchGate.
By embracing predictive analytics, organizations future-proof their strategies ensuring they stay ahead rather than merely reacting to change.
Charting the Future: Emerging Trends in Predictive Analytics
The predictive modeling landscape is evolving rapidly, with advancements in:
- Machine learning and AI: Deep learning and neural networks are pushing the boundaries of predictive accuracy. AI-driven models continuously improve, making predictions more precise and adaptable, as seen in Turing.
- Big data and cloud computing: With vast amounts of data available, cloud platforms like Google Cloud AI and AWS Machine Learning make predictive analytics accessible to businesses of all sizes. Read more from Bookdown.
- Ethical considerations: As predictive models influence hiring, lending, and law enforcement, concerns about bias and fairness have emerged. Transparent AI and ethical AI frameworks aim to mitigate these risks. Explore ethics via PMC.
Getting Started: Resources for Aspiring Data Scientists
For those looking to enter the world of predictive modeling, plenty of learning resources are available:
- Online courses and certifications: Platforms like Coursera, IBM Cognitive Class, and DataRobot offer training in predictive modeling fundamentals. Find a course on Coursera.
- Essential tools and software: Open-source tools like Python, R, and Dataiku are commonly used in predictive analytics. Explore tools at Dataiku.
- Building a career: From data science boot camps to advanced degrees, many paths lead to a thriving career in predictive analytics. Career guidance is provided by Indeed.
Predictive Modeling: The Data-Driven Engine of Tomorrow’s Economy
Predictive modeling isn’t just a futuristic concept it’s a fundamental driver of today’s economy. As businesses, governments, and healthcare institutions continue integrating AI-driven insights, the demand for skilled professionals in predictive analytics will only grow. Whether you’re a data scientist, a business leader, or just someone fascinated by the power of data, mastering predictive modeling offers limitless possibilities for innovation and impact.
You may also be interested in: Optimize FMCG Supply Chain with Digital Tools – StudioLabs
Ready to elevate your brand and transform your vision to digital solutions? Since 2003, StudioLabs has been trusted to help conceive, create, and produce digital products for the world’s most well-known brands to cutting-edge startups. Partner with StudioLabs and create something phenomenal. Let’s chat and experience StudioLabs in action with a complimentary session tailored to your business needs!