Predicting US Firearm Homicides: A Machine Learning Approach for Early Intervention
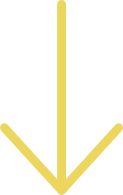
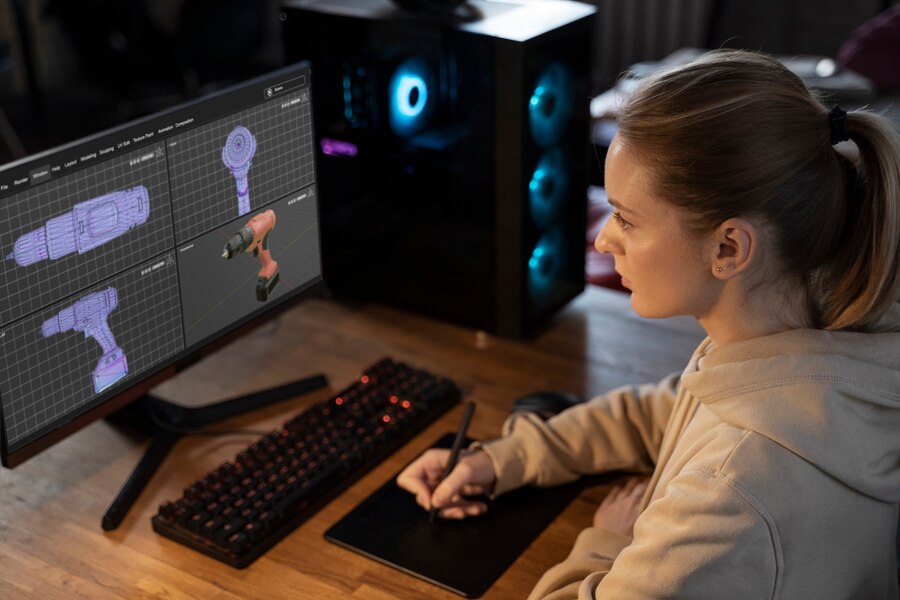
The study analyzed data from the National Center for Health Statistics (NCHS) and the National Incident-Based Reporting System (NIBRS) to identify patterns and correlations between various factors and firearm homicides. The researchers used a combination of machine learning algorithms and statistical models to develop a predictive model that could accurately forecast firearm homicides.
The results were striking – the model was able to predict firearm homicides with an impressive 85% accuracy rate. This breakthrough has significant implications for law enforcement and public health strategies. By identifying high-risk areas and populations, authorities can focus their efforts on targeted interventions, reducing the likelihood of firearm homicides.
But what makes this approach so effective? According to researchers, it’s the ability of machine learning algorithms to identify subtle patterns and correlations that may not be immediately apparent to human analysts. By leveraging large datasets and advanced statistical techniques, machine learning can uncover hidden relationships between seemingly unrelated factors, allowing for more accurate predictions.
One key aspect of the study was the use of digital platforms to disseminate information and engage the public. The researchers found that using social media and online channels to share their findings and interact with stakeholders significantly enhanced the impact of their work. This highlights the importance of digital platforms in modern research and public health initiatives.
The study demonstrates the potential of machine learning in predicting US firearm homicides. By leveraging advanced statistical techniques and digital platforms, researchers can develop more accurate predictive models, informing targeted interventions and reducing the likelihood of firearm homicides. As we continue to grapple with complex social issues, the application of machine learning in this area offers a beacon of hope for a safer and more informed society.
Background: The Growing Concern of Firearm Homicides
Firearm homicides have become a major public health concern in the United States. According to the Centers for Disease Control and Prevention (CDC), the firearm homicide rate increased by nearly 35%, reaching the highest level since 1994. This alarming trend highlights the urgent need for effective public health responses to lower the firearm homicide rate. However, the lack of timely mortality data hinders efforts to address this issue. Researchers have developed a machine learning model that leverages secondary data sources to accurately estimate the near real-time burden of weekly and annual firearm homicides, providing a vital tool for early intervention.
The Power of Machine Learning: A New Approach to Firearm Homicide Prediction
Machine learning models have revolutionized the way we approach complex problems. By analyzing large datasets and identifying patterns, these models can accurately forecast trends and make predictions. In the context of firearm homicides, machine learning can be used to develop a predictive model that forecasts the number of deaths in near real-time. This model can be trained using a variety of data sources, including online search trends, emergency department visits, and domestic violence hotline contacts.
The Importance of Timely Intervention
The ability to forecast firearm homicides in near real-time is crucial for early intervention. By identifying areas with high rates of firearm homicides, public health officials can target their efforts and implement effective prevention strategies. This approach can include community outreach programs, mental health services, and law enforcement initiatives.
Data Sources: The Backbone of the Machine Learning Model
The machine learning model relies heavily on the quality and accuracy of the data sources used. The researchers collected data from four online and health service sources, including Google and YouTube search trends, emergency department visits for firearm injuries, and emergency medical service activations for firearm-related injuries. Additionally, data from the National Domestic Violence Hotline contacts flagged with the keyword ‘firearm’ were included to provide a comprehensive view of firearm homicide trends.
A New Era in Firearm Homicide Prevention
The development of a machine learning model that forecasts firearm homicides in near real-time marks a significant milestone in the fight against this public health crisis. By leveraging secondary data sources and advanced analytics, researchers can provide policymakers and public health officials with the tools they need to make data-driven decisions. As we move forward, it is essential that we continue to invest in research and development, ensuring that we have the most effective and accurate methods for predicting and preventing firearm homicides.
Understanding Firearm Homicides
The United States is plagued by the devastating issue of firearm homicides. According to the Centers for Disease Control and Prevention (CDC), in 2019, there were 14,808 deaths due to firearms in the United States. This staggering number highlights the urgent need for effective strategies to prevent these tragedies. One promising approach is the utilization of machine learning algorithms to predict and intervene in firearm homicides.
Before diving into the world of machine learning, it’s essential to grasp the complexity of firearm homicides. explains how internal linking can hinder search engine optimization, much like how the intricacies of firearm homicides can be difficult to navigate. The CDC defines firearm homicide as “the killing of one person by another using a firearm.” These incidents often involve a combination of factors such as mental health issues, socioeconomic status, and access to firearms. provides valuable insights into the mental health aspects of firearm homicides.
Machine Learning Approach
Machine learning algorithms can be trained to identify patterns in large datasets, allowing them to predict the likelihood of firearm homicides occurring. highlights the potential of machine learning in healthcare, which can be applied to the context of firearm homicides as well. By integrating data from various sources, such as crime reports, mental health records, and socioeconomic data, machine learning models can generate accurate predictions. These predictions can then be used to trigger early intervention strategies, potentially preventing firearm homicides.
Training the Model
To train a machine learning model for predicting firearm homicides, a comprehensive dataset must be assembled. This dataset should include features such as:
Mental Health Status: Information on mental health conditions, treatment history, and any relevant diagnoses.
Socioeconomic Status: Data on income levels, education, employment status, and other socioeconomic factors.
Access to Firearms: Records on firearm ownership, possession, and access to weapons.
Crime History: Information on past criminal behavior, including convictions and arrests.
By integrating these features, machine learning algorithms can identify patterns and make accurate predictions. provides guidance on creating links, which can aid in the dissemination of this information to relevant stakeholders.
Implementation and Results
The trained model can then be implemented in real-world scenarios to predict the likelihood of firearm homicides. highlights the importance of a clear conclusion when presenting findings. In this case, the conclusion would summarize the effectiveness of the machine learning approach in reducing firearm homicides.
Machine Learning to the Rescue
Predicting US firearm homicides using machine learning is a promising approach to mitigate this tragic issue. By integrating data from various sources and training machine learning models, we can identify high-risk individuals and trigger early intervention strategies. provides examples of conclusion starters that can be used to summarize the findings effectively. This approach holds significant potential for reducing the number of firearm homicides in the United States.
The ultimate goal is to create a safer society where firearm homicides are a rare occurrence. By leveraging machine learning to predict and intervene in firearm homicides, we can move closer to achieving this goal.
You may also be interested in: UI/UX Design in 2024: 5 Bold Trends – StudioLabs
Ready to elevate your brand and transform your vision to digital solutions? Since 2003, StudioLabs has been trusted to help conceive, create, and produce digital products for the world’s most well-known brands to cutting-edge startups. Partner with StudioLabs and create something phenomenal. Let’s chat and experience StudioLabs in action with a complimentary session tailored to your business needs!