Machine Learning Models for Content Generation
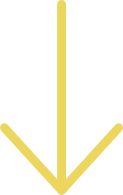
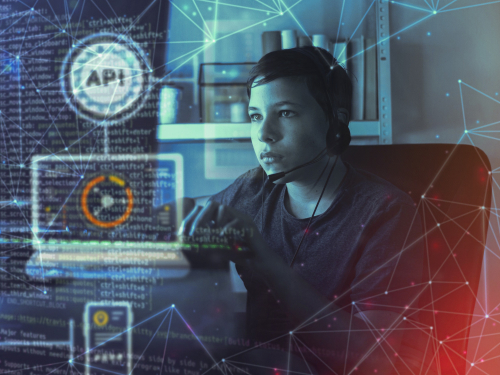
Quick Listen:
In recent years, the field of content generation has witnessed a transformative shift, largely driven by advancements in artificial intelligence (AI) and machine learning (ML). These technologies are not only enabling automation in various sectors but also reshaping the way content is created, optimized, and delivered. Machine learning models for content generation have revolutionized industries ranging from marketing and journalism to e-commerce and entertainment. By harnessing the power of natural language processing (NLP) and deep learning, AI algorithms can now create human-like text, generate creative content, and streamline writing processes in ways that were once unimaginable.
This article explores the key aspects of machine learning models for content generation, their capabilities, underlying technologies, and the impact they are having on industries worldwide.
The Rise of AI in Content Creation
Content generation is no longer confined to traditional methods such as manual writing, editing, or brainstorming. With AI, businesses and individuals can now produce high-quality content with remarkable speed and precision. Machine learning models, particularly those focused on natural language generation (NLG), are at the forefront of this transformation.
At the core of AI-driven content generation lies a branch of machine learning known as natural language processing (NLP). NLP empowers machines to understand, interpret, and produce human language, enabling them to generate text that is coherent, contextually accurate, and engaging. Over the years, NLP has evolved significantly, thanks to innovations in deep learning and neural networks, which have enhanced the ability of machines to comprehend complex linguistic patterns.
Machine learning models for content generation are designed to train on vast amounts of data, learning from examples of text, context, and meaning. These models leverage large datasets to understand the intricacies of language, including grammar, syntax, and semantics. As a result, AI can generate content that is not only grammatically correct but also stylistically rich and contextually relevant.
Natural Language Processing and Its Role
Natural language processing (NLP) plays a pivotal role in enabling machines to generate human-like content. NLP algorithms are designed to break down and analyze language, allowing machines to recognize patterns and relationships between words, phrases, and sentences. NLP techniques enable the model to understand things like tone, sentiment, and context, which are crucial when generating text that resonates with readers.
One of the most well-known examples of NLP in content generation is GPT (Generative Pretrained Transformer), developed by OpenAI. GPT is a language model that uses deep learning techniques to generate text. The model has been trained on massive amounts of text data from books, articles, websites, and other sources to generate coherent and contextually appropriate content. GPT can be fine-tuned for specific industries or writing styles, allowing it to create tailored content that meets the unique needs of businesses or individuals.
Other popular NLP models include BERT (Bidirectional Encoder Representations from Transformers), developed by Google, and T5 (Text-to-Text Transfer Transformer), which both enhance content generation by enabling deeper contextual understanding. These models are used for a wide range of tasks, including text summarization, translation, sentiment analysis, and, of course, content creation.
Deep Learning and Neural Networks
Deep learning, a subset of machine learning, is another key technology behind the success of machine learning models for content generation. Deep learning algorithms are based on artificial neural networks, which are designed to mimic the way the human brain processes information. These networks consist of layers of interconnected nodes, each responsible for processing specific aspects of input data. As the data passes through each layer, the network learns to recognize complex patterns and relationships.
In content generation, deep learning allows models to go beyond simple rule-based systems, enabling them to generate more creative and nuanced text. For example, deep learning models can be trained to recognize the structure of various writing styles, adapt to different tones of voice, and even incorporate humor or emotion into their generated content. This makes deep learning models incredibly versatile and capable of producing a wide range of content, from news articles to marketing copy, social media posts, and even poetry.
Recurrent Neural Networks (RNNs) and Long Short-Term Memory (LSTM) networks are examples of deep learning techniques often used in content generation. These models are designed to process sequential data, making them particularly well-suited for generating coherent text that follows a logical progression.
Training Machine Learning Models for Content Generation
Training machine learning models for content generation involves feeding the system with large datasets, allowing it to learn from patterns and correlations within the data. The quality of the dataset plays a significant role in determining the performance of the model. For content generation, training data often includes a diverse array of text sources such as articles, books, websites, product descriptions, and more.
The training process typically involves two main stages: pretraining and fine-tuning. In the pretraining stage, the model is exposed to a massive dataset to learn the general structures and patterns of language. During fine-tuning, the model is exposed to more specific data related to the intended use case, such as legal language, technical writing, or marketing copy. Fine-tuning ensures that the model produces content that aligns with the specific goals and tone required.
One of the challenges in training machine learning models for content generation is ensuring that the model can generate text that is not only relevant but also accurate and free from biases. Models are trained to avoid harmful language, false information, and stereotypes, though challenges remain in achieving complete reliability. As machine learning models evolve, efforts to improve the quality of generated content continue.
Benefits of AI in Content Generation
- Speed and Efficiency: One of the most significant advantages of machine learning models in content generation is the speed at which they can produce text. AI-driven systems can generate large volumes of content in a fraction of the time it would take a human writer. This is particularly useful in industries like e-commerce, where product descriptions need to be created quickly, or digital marketing, where social media content and blog posts are needed on a regular basis.
- Personalization: AI models can be trained to tailor content to specific audiences, taking into account factors such as demographic data, user preferences, and behavior. This ability to personalize content increases engagement and ensures that the right message is delivered to the right people.
- Consistency and Scalability: Machine learning models can maintain a consistent tone and style across all generated content. This is particularly important for brands looking to maintain a unified voice across various channels. Additionally, AI models can scale content production, allowing businesses to meet the demands of high-volume content creation without sacrificing quality.
- Cost-Effectiveness: AI-driven content generation can reduce the need for human writers, editors, and content creators, leading to cost savings. While human creativity and input are still essential in many areas, AI can handle repetitive or data-driven tasks, freeing up human resources for more strategic work.
- Creative Exploration: AI models can also assist in the creative process. They can generate ideas, provide inspiration, or even write the first draft of a piece, leaving human writers to refine and polish the content. This can help overcome writer’s block and foster new creative approaches.
Challenges and Considerations
While the benefits of machine learning models for content generation are clear, there are still challenges to address. For instance, ensuring that AI-generated content is free of biases, inaccurate information, or harmful stereotypes remains a priority. Furthermore, while AI can generate human-like text, it still lacks the deep understanding and emotional intelligence that human writers bring to the table.
The ethical implications of AI-generated content also raise concerns, particularly in areas like journalism, where accuracy and transparency are paramount. Ensuring that AI-generated content is clearly labeled and doesn’t mislead readers is critical in maintaining trust.
Expand at Scale
Machine learning models for content generation have proven to be powerful tools in transforming the way content is created, optimized, and delivered across industries. By leveraging technologies such as natural language processing and deep learning, AI can generate high-quality, personalized, and relevant content at scale. As machine learning models continue to evolve, their capabilities will only expand, further disrupting traditional content creation methods and providing businesses with new opportunities to engage audiences in creative and innovative ways.
You may also be interested in: AI Prototyping: Turning Ideas Into Breakthrough Products – StudioLabs
Ready to elevate your brand and transform your vision to digital solutions? Since 2003, StudioLabs has been trusted to help conceive, create, and produce digital products for the world’s most well-known brands to cutting-edge startups. Partner with StudioLabs and create something phenomenal. Let’s chat and experience StudioLabs in action with a complimentary session tailored to your business needs!