5 Steps to Integrate AI Like a Pro: A Practical Guide for Business Leaders
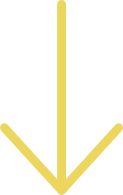
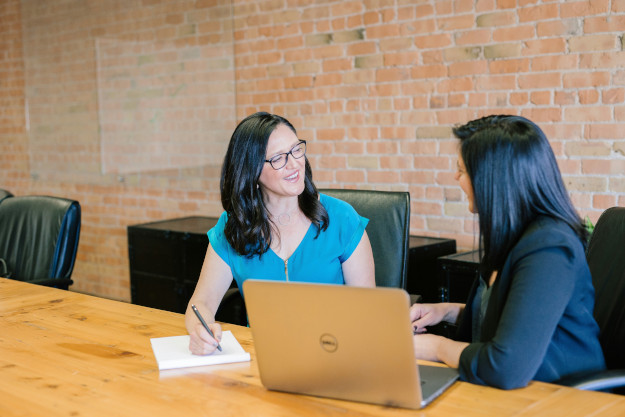
Integrating artificial intelligence (AI) into business operations has become a critical strategy for staying competitive in today’s market. However, for many business leaders, the prospect of implementing AI can be daunting. Let’s explore the essential steps and best practices for integrating AI seamlessly into your organization. From identifying the right AI solutions to overcoming implementation challenges, we will provide you with the knowledge and tools needed to navigate the world of AI with confidence. Whether you’re a seasoned executive or a budding entrepreneur, this guide will help you harness the power of AI like a pro.
According to Jared Spataro, Harvard Business Review, “And while AI holds the promise of transforming work and giving organizations a competitive advantage realizing those benefits isn’t possible without a culture that embraces curiosity, failure, and learning.” Staying competitive in the business world requires a strategic embrace of AI. The ability to harness the power of AI not only streamlines processes but also enhances decision-making, customer experiences, and overall operational efficiency. AI integration plays a critical role in maintaining competitiveness in today’s fast-paced and data-driven business environment.
The Artificial Intelligence market attained a size of USD 428.00 Billion in 2022, and there are projections indicating a substantial increase to USD 2,025.12 Billion by 2030. The growth pace of the Artificial Intelligence Market is remarkable, with an anticipated Compound Annual Growth Rate (CAGR) of 21.6% throughout the forecast period from 2023 to 2030.
Understanding AI in Business
A. AI and its applications in different industries
To effectively integrate AI into business operations, it’s crucial to understand what AI is and its diverse applications. This section will provide a concise definition of AI, emphasizing its ability to simulate intelligent human behavior. Furthermore, it will explore real-world applications across various industries, such as healthcare, finance, manufacturing, and customer service. Demonstrating the versatility of AI sets the stage for business leaders to envision its potential impact on their specific domains.
B. AI integration for business operations and decision-making
The adoption of AI can yield a multitude of benefits for businesses. This section will outline how AI integration enhances operational efficiency, accelerates decision-making processes, and contributes to cost reduction. It will delve into specific examples, showcasing how AI-driven insights lead to more informed and strategic choices. By understanding the potential advantages, business leaders can better appreciate the tangible impact AI can have on their organizations.
C. Common misconceptions about AI that business leaders should be aware of
Misconceptions surrounding AI can hinder its adoption. Common myths and misconceptions, are the fear of job displacement, the idea that AI is only for large enterprises, or the misconception that AI is a one-size-fits-all solution. Dispel these myths allows business leaders to approach AI adoption with a clearer understanding, facilitating smoother integration and maximizing the benefits for their specific business needs. Understanding the potential challenges and debunking misconceptions is crucial for informed decision-making during the AI integration process.
Assessing Your Business Needs
A. Identifying areas where AI can add value
Before diving into AI integration, business leaders must identify specific areas where AI can add the most value. Pinpoint processes that could benefit from automation, data analysis, or enhanced decision-making capabilities. By understanding the unique challenges and opportunities within their organization, leaders can tailor AI solutions to address specific needs and goals.
B. Conducting a thorough analysis of current business processes
A critical step in AI integration is conducting a comprehensive analysis of existing business processes. This involves mapping out workflows, identifying bottlenecks, and assessing data flow. By gaining a deep understanding of current processes, business leaders can pinpoint pain points and areas ripe for improvement through AI implementation. Provide a structured approach to conducting a thorough analysis, ensuring that AI solutions are aligned with the organization’s objectives and challenges.
C. Evaluating the readiness of the organization for AI integration
AI integration requires not only technological readiness but also organizational preparedness. Evaluate the company’s readiness for AI adoption. Factors such as data quality, IT infrastructure, employee skills, and organizational culture will be explored. By addressing potential barriers and assessing the organization’s capacity for change, leaders can proactively plan for a successful AI integration, minimizing disruptions and maximizing the chances of success.
Creating a Cross-Functional AI Team
A. Importance of collaboration between IT, data science, and business departments
Successful AI integration requires seamless collaboration between different departments within an organization. Emphasize the significance of breaking down silos and fostering collaboration between IT, data science, and business units. Highlighting the interconnected nature of these functions, it will stress the need for open communication channels and shared goals to ensure that AI projects align with the overall business strategy.
B. Building a diverse team with a mix of technical and business expertise
Diversity in skills and perspectives is key to the success of an AI integration team. Build a team with a diverse skill set, including technical expertise in areas like machine learning, data engineering, and software development, as well as business acumen to understand the strategic objectives of the organization. Emphasizing the importance of a multidisciplinary team will help ensure a holistic approach to AI integration that addresses both technical and business requirements.
C. Defining roles and responsibilities within the AI integration team
To avoid confusion and streamline the AI integration process, it’s crucial to clearly define roles and responsibilities within the team. Outline key roles, such as data scientists, AI engineers, project managers, and business analysts, and elaborate on their specific responsibilities. By establishing a well-defined structure, business leaders can create a cohesive team that operates efficiently and collaboratively, driving the AI integration process forward with a clear understanding of each team member’s contribution.
Selecting the Right AI Solutions
A. Researching and understanding various AI technologies
Before making decisions about AI integration, business leaders need to familiarize themselves with the diverse range of AI technologies available. Get a overview of different AI technologies such as machine learning, natural language processing, and computer vision. It will emphasize the importance of understanding the strengths, limitations, and practical applications of each technology, enabling business leaders to make informed choices aligned with their specific business needs.
B. Evaluating AI vendors and solutions based on business requirements
Selecting the right AI vendor and solution is a critical step in the integration process. Develop a process of evaluating vendors, emphasizing the need to align potential solutions with specific business requirements. Discuss factors such as the vendor’s reputation, expertise, support services, and the flexibility of their solutions. By conducting a thorough evaluation, business leaders can choose a vendor that not only provides cutting-edge technology but also meets the unique needs of their organization.
C. Considering scalability, security, and compatibility with existing systems
Scalability, security, and compatibility are crucial considerations when integrating AI solutions into existing business processes. This section will delve into the importance of choosing AI solutions that can scale alongside the organization’s growth. It will also address the paramount concern of ensuring the security of sensitive data and the need for compatibility with existing IT infrastructure. By focusing on these aspects, business leaders can mitigate potential challenges and lay the foundation for a seamless integration that aligns with long-term business goals.
Data Preparation and Quality
A. Ensuring data accuracy, completeness, and relevance
The success of AI initiatives heavily relies on the quality of the data used. This section will emphasize the importance of ensuring that data is accurate, complete, and relevant for the intended AI applications. It will guide business leaders through processes for data profiling, validation, and verification, helping them understand the significance of maintaining high-quality data to achieve reliable and meaningful insights from AI systems.
B. Implementing data governance and security measures
To maintain data integrity and protect sensitive information, businesses must implement robust data governance and security measures. This section will explore the establishment of data governance policies, access controls, and encryption methods. Emphasizing the importance of compliance with data protection regulations, it will guide business leaders in creating a secure environment that fosters trust in AI applications and safeguards the organization against potential risks.
C. Cleaning and pre-processing data for optimal AI performance
Raw data often requires cleaning and pre-processing to enhance its suitability for AI applications. This section will delve into the importance of data cleaning techniques, including handling missing values, addressing outliers, and normalizing data. It will also highlight the role of feature engineering in optimizing data for machine learning algorithms. By understanding the significance of preparing data for AI, business leaders can ensure that their systems operate with maximum efficiency, producing accurate and reliable results.
Pilot Testing and Iterative Implementation
A. Starting with a small-scale AI implementation to test feasibility
Embarking on a small-scale pilot is a prudent approach to test the feasibility of AI integration. This section will guide business leaders through the process of selecting a specific use case or business process for the initial implementation. By starting small, organizations can assess the practicality and impact of AI in a controlled environment, minimizing risks and allowing for adjustments before scaling up.
B. Gathering feedback and making necessary adjustments
Feedback is a crucial element in the iterative implementation of AI solutions. This section will emphasize the importance of collecting feedback from end-users, stakeholders, and the AI implementation team. It will guide business leaders on how to analyze feedback effectively and make necessary adjustments to improve system performance, user experience, and alignment with business objectives. This iterative feedback loop ensures that AI solutions evolve to meet the evolving needs of the organization.
C. Scaling up the AI integration gradually based on successful outcomes
Once the pilot phase demonstrates successful outcomes and necessary adjustments have been made, business leaders can proceed to scale up AI integration across broader business functions. This section will provide a roadmap for a gradual and strategic expansion, considering factors such as resource allocation, organizational readiness, and the identification of new use cases. By scaling up based on proven success, organizations can maximize the impact of AI while minimizing potential disruptions to ongoing operations.
Training and Upskilling
A. Providing training programs for employees on AI technologies
The successful integration of AI into business operations requires a skilled and knowledgeable workforce. This section will guide business leaders in developing comprehensive training programs to equip employees with the necessary skills and understanding of AI technologies. From basic AI literacy to specialized skills such as data science and machine learning, investing in employee training ensures that the workforce is well-prepared to embrace and contribute to the AI-driven transformation.
B. Encouraging a culture of continuous learning and adaptation
The dynamic nature of AI technology necessitates a culture of continuous learning within the organization. This section will emphasize the importance of fostering an environment that encourages employees to adapt, learn, and stay abreast of evolving AI trends. It will provide strategies for promoting ongoing education, including mentorship programs, online courses, and knowledge-sharing initiatives. By instilling a culture of continuous learning, businesses can ensure that their workforce remains agile and responsive to the changing demands of AI integration.
C. Addressing concerns and fostering a positive attitude towards AI among the workforce
Employee concerns and reservations about AI adoption are common and can hinder the integration process. This section will address potential concerns, such as job displacement fears and skepticism about AI technologies. It will provide guidance on transparent communication, highlighting the collaborative nature of AI-human partnerships and the potential for AI to enhance job roles rather than replace them. Fostering a positive attitude towards AI among the workforce is crucial for successful integration and employee buy-in.
Monitoring and Optimization
A. Implementing monitoring tools for AI performance
Effective AI integration requires continuous monitoring to ensure optimal performance. This section will guide business leaders in implementing monitoring tools and systems to track the performance of AI applications. It will cover key metrics, such as accuracy, latency, and system responsiveness. By establishing a robust monitoring framework, organizations can promptly identify and address issues, ensuring the reliability and effectiveness of their AI solutions.
B. Regularly reviewing and optimizing AI algorithms and models
AI algorithms and models require ongoing attention to maintain relevance and effectiveness. This section will emphasize the importance of regularly reviewing and optimizing AI models to adapt to changing business dynamics and data patterns. It will provide insights into techniques such as retraining models, updating algorithms, and incorporating new data sources. By prioritizing continuous improvement, organizations can ensure that their AI systems evolve to meet the ever-changing demands of their business environment.
C. Staying informed about advancements in AI to keep systems up-to-date
The field of AI is dynamic, with rapid advancements and breakthroughs. Business leaders must stay informed about the latest developments to keep their AI systems up-to-date. This section will guide leaders on how to establish channels for staying informed, including participation in industry conferences, networking with AI experts, and subscribing to relevant publications. By staying abreast of advancements, organizations can leverage cutting-edge technologies and maintain a competitive edge in their industry.
Lay the Foundation
Successful AI integration is not just a short-term solution; it lays the foundation for long-term benefits. This section will underscore the potential advantages, including increased operational efficiency, enhanced decision-making, and improved competitiveness. By emphasizing the transformative power of AI when integrated effectively, business leaders are encouraged to view AI adoption as a strategic investment with far-reaching positive impacts.
Ready to elevate your brand and transform your vision to digital solutions? Since 2003, StudioLabs has been trusted to help conceive, create, and produce digital products for the world’s most well-known brands to cutting-edge startups. Partner with StudioLabs and create something phenomenal. Let’s chat and experience StudioLabs in action with a complimentary session tailored to your business needs!